Why Is Metadata Important? Understanding Its Types and Uses
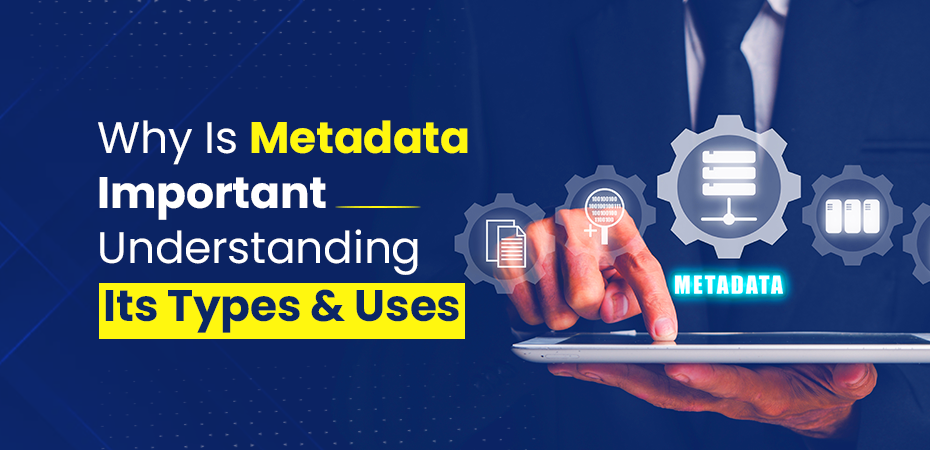
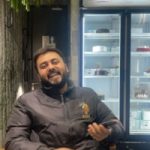

Metadata, often described as “data about data”, is crucial in the field of data management. It provides essential information that helps to contextualize, organize, and manage data effectively. Understanding why metadata is important can significantly enhance how organizations handle their data assets.
The significance of metadata extends across various aspects of data management:
- Contextual Understanding: Metadata provides context, detailing the source, type, owner, and relationships with other datasets.
- Enhanced Discoverability: Facilitates the sharing and finding of data across platforms.
- Governance and Compliance: Ensures adherence to policies and regulations like GDPR.
- Operational Efficiency: Streamlines dataset identification based on attributes.
The purpose of this article is to explore the different types of metadata and their uses. By understanding these aspects, organizations can better manage their data, ensuring it remains a valuable asset for decision-making and operational efficiency.
Here, in this blog, you will learn about meta data definition along with its different types and metadata examples.
Understanding Metadata
The true meaning of metadata, often referred to as data about data, plays a crucial role in the effective management and utilization of data. To dive deeper into its significance, it’s essential to grasp a detailed metadata definition and understand its various facets.
Definition of Metadata
While defining metadata in simple terms, it is information that describes other data. This meta-information can include attributes such as:
- Title
- Author
- Creation Date
- File Size
- Format
For instance, a digital photograph’s metadata might include details like the camera model, exposure settings, date taken, and GPS coordinates.
Distinction Between Data and Metadata
It is vital to distinguish between data and metadata meaning:
- Data: The actual content or primary information (e.g., the text of an email).
- Metadata: Information about that content (e.g., sender, recipient, date sent).
While data represents the core substance, metadata provides context, making it easier to understand and manage the underlying data. However, the metadata class is used across multiple tasks and tables.
Importance of ‘Data About Data’
The phrase ‘data about data’ encapsulates metadata’s essence. It enriches datasets by offering:
- Contextual Clarity: Metadata analysis helps comprehend the origin, structure, and relevance of data.
- Efficient Organization: By categorizing and tagging datasets, meta data ensures streamlined storage and retrieval.
- Enhanced Discoverability: Facilitates easier search and access across platforms.
Example: In a digital library setting, metadata for a book might encompass the title, author, publication year, genre, ISBN number, and summary. This meta information not only aids cataloging but also simplifies user searches.
Understanding these fundamental aspects underscores why metadata is indispensable in modern data ecosystems.
The Importance of Metadata in Data Management
Contextual Understanding
Metadata is essential for understanding data in context. It provides important details such as where the data came from, what type it is, who owns it, and how it relates to other datasets. This information transforms data from being just a random collection of numbers and text into something meaningful and valuable. For example, knowing where a dataset originated or when it was last modified can greatly influence how we interpret and use that data.
Data Sharing and Discoverability
One of the advantages of metadata is its ability to improve data sharing and discoverability. By summarizing key information about datasets, meta deta makes it easier to find and use data across different platforms and organizations. Think of it like a library catalog: just as author names, publication dates, and genres help us locate books effortlessly, metadata does the same for datasets.
Improved Data Quality
Metadata is crucial for maintaining high-quality data. It provides insights into how the data was collected, how accurate it is, and other indicators of quality. This transparency builds trust among users who rely on this data for their work, leading to better decision-making.
Interoperability
Another significant benefit of metadata is its role in ensuring interoperability between systems. When different software applications use standardized formats for metadata (such as XML or JSON), they can easily understand each other’s data files without any compatibility issues. This means that organizations can integrate their systems seamlessly and share data more effectively.
Governance and Compliance
In today’s world where regulations like GDPR impose strict rules on how we handle personal information, metadata becomes even more critical. It helps us classify sensitive data, control who has access to it, and comply with legal requirements. For instance, by tagging personal information with specific metadata fields, organizations can efficiently manage consent records and fulfill their obligations under these regulations.
Decision-Making Support
Metadata also enhances analytical processes by providing the necessary context for interpreting data accurately. When analysts have access to background details—such as how the data was collected or what variables are included—they can make better decisions based on that information. This contextual understanding is invaluable in various fields like market analysis or scientific research.
Operational Efficiency
Lastly, metadata improves operational efficiency by making it easier to identify datasets based on specific attributes such as date or category. With automated processes in place, large amounts of data can be quickly sorted through using predefined metadata tags to find relevant datasets. This not only saves time but also reduces manual effort in managing our data assets.
Understanding these aspects highlights why integrating robust meta-data practices into your organization’s data management strategy is crucial.
Types of Metadata and Their Uses
Understanding the different types of metadata is essential to appreciate its full potential in data management. Each type serves unique purposes, offering distinct advantages for various applications.
1. Descriptive Metadata
Descriptive metadata provides details about the content of a resource. It includes:
- Title: The name of the dataset or resource.
- Abstract: A brief summary explaining what the dataset is about.
This type of metadata is crucial for identifying and discovering resources. For example, a library catalog uses descriptive metadata to help users find books based on titles and summaries.
2. Structural Metadata
Structural metadata describes how different components of a resource are organized. Examples include:
- Tables of Contents: An outline or listing of chapters and sections within a document.
- Indexes: Detailed lists that direct users to specific parts of a resource.
This type helps in understanding the internal structure, making it easier to navigate complex datasets or documents.
3. Administrative Metadata
Administrative metadata encompasses management-related information, such as:
- Creation Date: When the data was created.
- Access Rights: Who can access or modify the data.
These details support effective data governance and management practices by tracking data provenance and access permissions.
4. Reference Metadata
Reference metadata offers insights into the contents or quality of statistical data. It includes:
- Methodology Descriptions: Information on how data was collected or processed.
- Quality Assessments: Evaluations of data reliability and accuracy.
This type is valuable for ensuring that statistical analyses are based on high-quality, well-documented data.
5. Statistical Metadata
Statistical metadata provides processing details regarding statistical datasets:
- Data Processing Steps: Documentation of how raw data was transformed into final statistics.
- Variable Definitions: Explanations of statistical measures used in the dataset.
This type aids analysts in understanding the workflow behind statistical results, ensuring transparency and reproducibility.
6. Business Metadata
Business metadata defines rules related to business operations and specific data elements. Examples include:
- Business Rules: Conditions that must be met for data to be considered valid.
- Data Element Definitions: Clarifications on what each piece of business data represents.
This type helps align data with business objectives, ensuring that datasets meet organizational standards and requirements.
7. Operational Metadata
Operational metadata contains technical details about resource versioning:
- Version Numbers: Identifiers for different iterations of a dataset or document.
- Change Logs: Records of modifications made over time.
This information supports version control, making it easier to track changes and manage updates effectively.
8. Legal Metadata
Legal metadata comprises information regarding legal obligations associated with the data:
- Compliance Requirements: Specific regulations that govern how data must be handled (e.g., GDPR).
- Usage Restrictions: Limitations on how the data can be used or shared.
By addressing legal considerations, this type ensures that organizations adhere to relevant laws and regulations, reducing risks associated with non-compliance.
Understanding these types helps leverage metadata’s full potential across multiple tasks and tables. Each category plays a vital role in enhancing usability, governance, quality assurance, and operational efficiency within any organization.
Best Practices for Effective Metadata Management
Effective metadata management is critical for ensuring data usability and integrity. Implementing the following best practices for metadata strategy can significantly enhance data handling:
1. Establishing Standardized Naming Conventions
Consistency in naming conventions helps in maintaining uniformity across datasets. This standardization facilitates easier data retrieval and reduces confusion, particularly when dealing with large volumes of information.
2. Creating Customized Templates for Different Types of Datasets
Customized templates ensure that all necessary metadata elements are captured for various dataset types. These templates can be tailored to specific industries or organizational needs, streamlining the metadata entry process and improving data quality.
3. Regular Audits to Ensure Accuracy and Completeness
Conducting regular audits helps in identifying discrepancies or gaps in metadata. This ensures the metadata remains up-to-date and accurate, which is essential for reliable data analysis and decision-making.
4. Training Staff on the Importance and Use of Metadata
Educating staff about the significance of metadata and how to effectively use it enhances overall data management practices. Training sessions can cover topics such as metadata creation, maintenance, and best practices, fostering a culture of data stewardship within the organization.
By incorporating these strategies, organizations can better manage their data assets, leading to improved efficiency and more informed decision-making processes.
Tools for Improving Metadata Quality
Effective metadata management tools are essential for maintaining high data quality and usability. You might be thinking which of the following accurately describes metadata? Two notable tools in this space include Atlan and Opendatasoft.
Popular Tools
1. Atlan
A collaborative workspace designed for data teams, offering features such as automated metadata tagging, data lineage, and governance controls. Atlan integrates seamlessly with various data sources, enhancing the accessibility and discoverability of metadata.
2. Opendatasoft
Known for its user-friendly interface, Opendatasoft aids in cataloging, enriching, and sharing datasets. It provides robust search functionalities and easy-to-use APIs that facilitate data integration across platforms.
Features to Look For
When selecting a metadata management tool, consider the following features:
- Integration Capabilities: Ensure the tool can integrate with existing systems and data sources for seamless metadata management.
- Automated Tagging: Look for tools that offer automated tagging and classification to reduce manual effort and increase accuracy.
- Data Lineage Tracking: This feature helps in understanding the origins and transformations of data, crucial for maintaining data integrity.
- User-Friendly Interface: An intuitive interface makes it easier for users to navigate and manage metadata effectively.
- Governance Controls: Strong governance features help in enforcing compliance with policies and regulations.
Selecting the right tool enhances your ability to manage metadata effectively, ensuring your data remains organized, accessible, and reliable.
Real-World Examples of Effective Metadata Usage
Understanding the practical applications of metadata can provide insights into its value in data management. Here, we delve into how industry giants like Google and Amazon Web Services (AWS) leverage metadata to enhance their operations.
Google’s search engine is a prime example of how metadata can be effectively used to improve data discoverability and usability. Here are some key aspects to discover what is SEO meta data :
- Search Engine Optimization (SEO): Google’s algorithms rely heavily on metadata such as meta tags, titles, and descriptions to rank web pages. This metadata SEO helps users find relevant content quickly.
- Google Photos: Metadata in Google Photos includes information like date, time, location, and even facial recognition details. This enables efficient organization, search, and retrieval of images.
Example: Searching for “beach vacation 2022” in Google Photos will pull up images tagged with those terms, thanks to metadata.
Amazon Web Services (AWS)
AWS uses metadata extensively to manage its vast cloud infrastructure and services. Some notable applications include:
- Amazon S3 (Simple Storage Service): AWS S3 utilizes metadata for organizing and managing data stored in buckets. Metadata tags help in categorizing objects based on attributes like project name or department.
- json { “Key”: “project”, “Value”: “AI Research” }
- Data Governance: AWS Lake Formation employs metadata for implementing data governance policies. This ensures compliance with regulations like GDPR by tagging sensitive data appropriately.
Example: Labeling datasets containing personal information with specific tags to enforce access controls.
Conclusion
Metadata is an indispensable element in the realm of data management, offering a framework that enhances contextual understanding, data sharing, discoverability, and overall data quality. By providing detailed information about the source, type, ownership, and relationships of data, meta data meaning supports interoperability, governance, compliance, and informed decision-making.
Effective metadata management practices, such as standardized naming conventions and regular audits, coupled with advanced tools like those from Google and AWS, further bolster operational efficiency. Despite challenges such as lack of awareness and inconsistent standards, the future of metadata management looks promising with advancements in AI and machine learning poised to revolutionize the field.